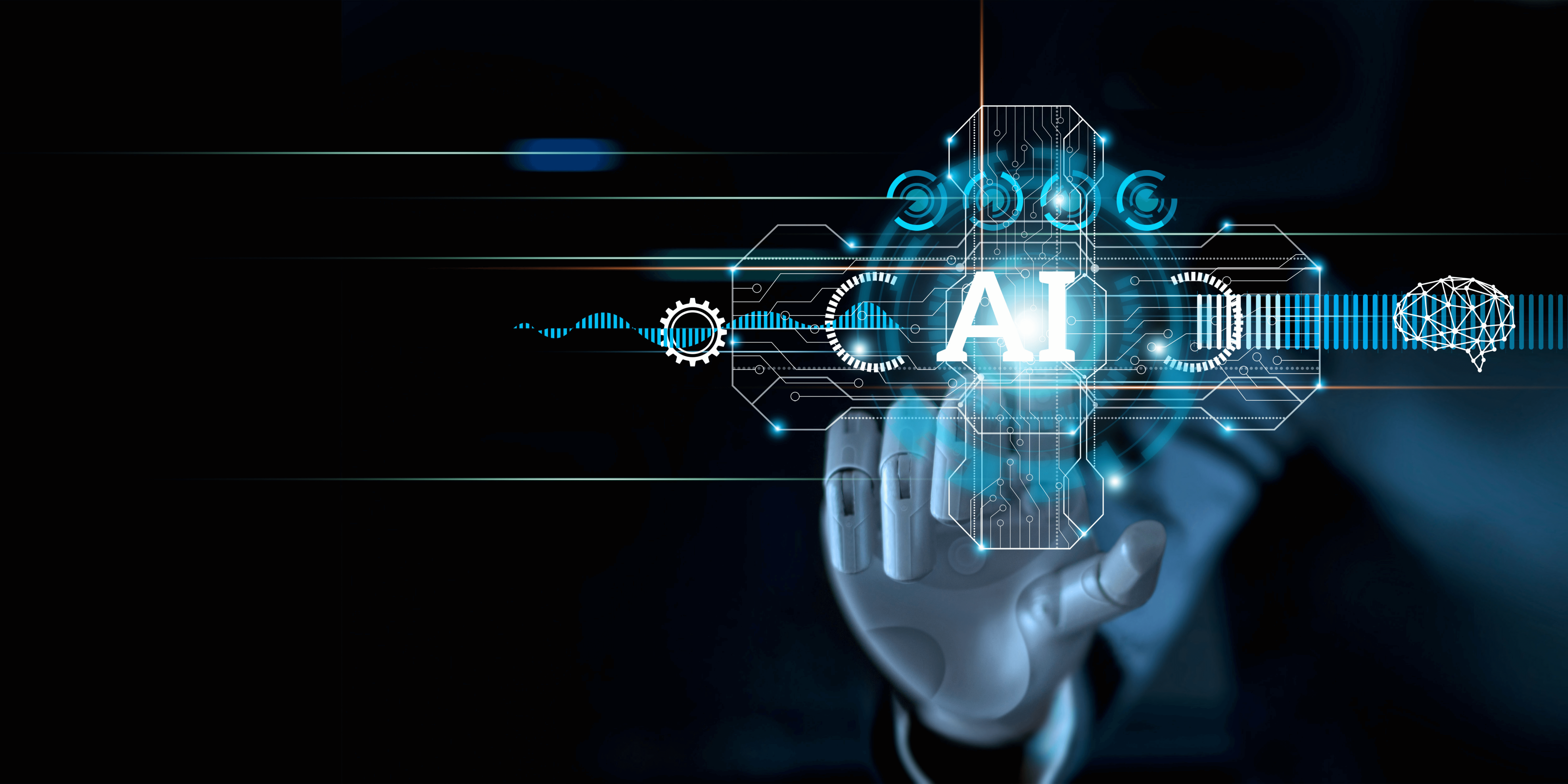
Let's start from point zero -
What is a Machine Learning (ML) model?
In simple terms, an ML model is nothing but a mathematical engine of AI that has a voracious appetite for data to help find patterns and make predictions faster than humans can. The quality of data you feed it will determine the quality and accuracy of predictions it makes.
What is ML modernization?
ML modernization is all about upgrading your existing ML models to make them more efficient and effective. It involves using the latest technologies and techniques to improve the accuracy and speed of your ML models.
The need for ML modernization:
When you shop for a car or a phone, you always think about the latest models to buy. Why so? Reasons can vary from better efficiency, storage, security, newer features, etc. So, when you run a business, why should you not desire the same for the ML models you have built? ML modernization has become crucial to harness the full potential of ML technology, for organizations to stay competitive, make more informed decisions, and provide better user experience. Some of the reasons why it has become an impending need are:
- Adapting to the ever-growing and changing nature of data: ML models are fueled by data. Success in the implementation of AI and ML strategies can depend on your organization's ability to harvest massive quantities of data from a large and disparate group of sources, i.e., your customers. However, the real difficulty lies in ensuring that the data collected is representative of your entire customer base. From the time you built your ML models, data must have grown enormously, and the nature of data being collected must have also changed drastically. Additionally, care must be exercised continuously so that there is no human bias creeping from your data. The selection criteria of data must be chosen wisely for feeding into ML models.
- Advancement and integration with new technologies: To improve the performance of ML models, one has to integrate them with the latest relevant technologies like edge computing, advancements in cloud computing, live streaming of data techniques, IoT, etc., to be able to operate in a distributed environment and gather data from newer sources.
- Cost optimization: Modernization can lead to cost optimization by reducing computational resource requirements, improving energy efficiency, or optimizing model architectures. By optimizing ML systems, businesses can achieve better results with fewer resources, resulting in cost savings.
- Adherence to regulatory compliances: Compliance with data protection regulations, such as the General Data Protection Regulation (GDPR), becomes easier when ML systems are modernized. Incorporating privacy-enhancing techniques, anonymization methods, or secure data handling practices ensures compliance with legal and ethical requirements.
When is the right time for building ML models or ML modernization?
There is no perfect time to start your journey of building ML models. If you overanalyze whether your data is perfect or not, you will never be able to build ML models as these are continuous processes. Yes, the more data you feed your models, the better they will be. But you must get your models out of the door and into the real world at some point for them to begin delivering results. Beginning with the data you have available is better than not beginning at all. Data is a gold mine only when it is being used; otherwise, it will be useless.
If you're interested in building new ML models or modernizing your existing ones, we'd love to hear from you! Contact us today at [email protected] to start your ML modernization journey and unlock the full potential of AI and ML for your business. Happy gold mining!