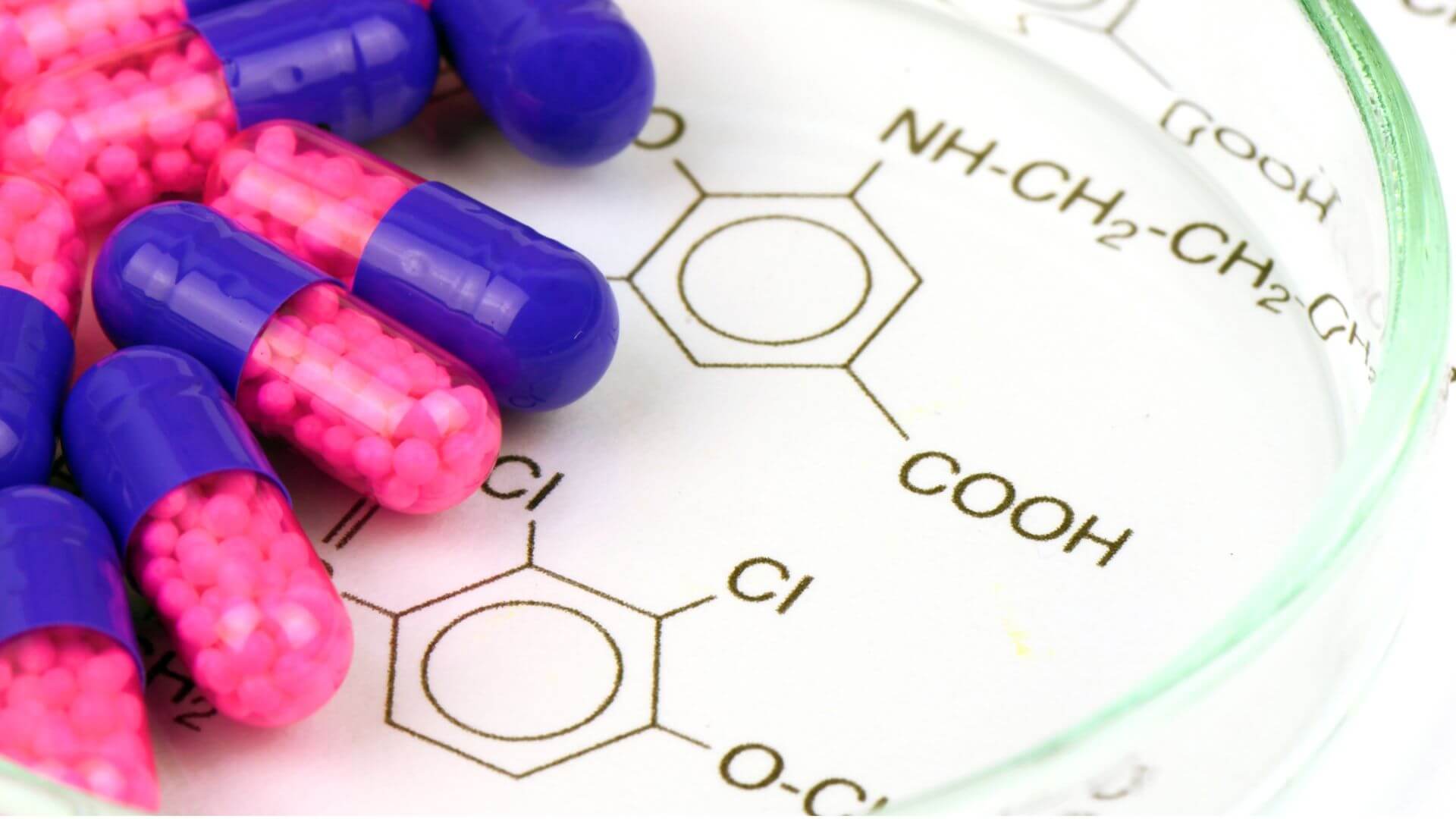
Innovations in Pharma: How AI and ML are Reshaping the Future of Medicine

Shubham Khandelwal
Senior Business Analyst
Did you know?
It can take up to 12 years to bring a new drug to market, from research and development to clinical trials and regulatory approval.
The cost of developing a new drug can vary widely but estimates suggest that it can cost anywhere from $1 billion to $2.6 billion to bring a single drug to market, depending on factors such as the complexity of the disease being targeted and the length of clinical trials.
The success rate for drug candidates that enter clinical trials is typically low, with only about 10-15% of candidates ultimately receiving regulatory approval.
The majority of drug candidates fail during preclinical testing, which is typically the first step in the drug development process. Only about 5% of drug candidates that enter preclinical testing make it to clinical trials.
Drug Discovery Lifecycle
Basic Research: The drug discovery process begins with basic research, which involves identifying a biological target that is implicated in a disease. Researchers then screen large numbers of chemical compounds to find ones that interact with the target.
Pre-Clinical Trials: they are generally called preclinical trials Once a promising drug candidate has been identified, it must undergo non-clinical trials to evaluate its safety and efficacy in animals. This stage includes testing for toxicity, pharmacokinetics, and pharmacodynamics.
Phase 1 to 3 Clinical Trials:
- Phase 1 Clinical Trials:
Phase 1 trials are the first step in evaluating the safety and tolerability of a drug candidate in humans. These trials typically involve a small group of healthy volunteers, usually ranging from 20 to 100 participants. The primary focus is to assess the drug's pharmacokinetics (how the drug is absorbed, distributed, metabolized, and excreted), pharmacodynamics (how the drug interacts with the body), and determine the safe dosage range.
- Phase 2 Clinical Trials:
Once a drug candidate successfully passes Phase 1 trials, it moves on to Phase 2 trials, which involve a larger number of patients. These trials aim to assess the drug's efficacy and further evaluate its safety profile. Phase 2 trials can involve several hundred participants and are typically divided into two or more groups. Patients in these groups may receive different dosages or formulations of the drug, or they may be compared to a control group receiving a placebo or an existing standard treatment. The results obtained from Phase 2 trials help determine the optimal dosing regimen and provide initial evidence of the drug's effectiveness.
- Phase 3 Clinical Trials:
Phase 3 trials are the final stage of clinical testing before seeking regulatory approval. They involve a larger patient population, often ranging from several hundred to several thousand participants, and are conducted across multiple clinical sites. Phase 3 trials aim to further confirm the drug's effectiveness, monitor side effects, and collect additional safety data in a more diverse patient population. These trials are crucial in providing robust evidence of the drug's benefits and risks, as well as determining the appropriate usage guidelines and potential adverse reactions.
Application Approval Marketing: If a drug candidate successfully completes clinical trials, the drug sponsor can submit a New Drug Application (NDA) or Biologics License Application (BLA) to the regulatory agency for approval. If the application is approved, the drug can be marketed to patients.
Post-Marketing Surveillance: Once a drug is on the market, post-marketing surveillance is conducted to monitor its safety and efficacy in real-world settings. This includes ongoing pharmacovigilance activities, such as monitoring for adverse events and drug interactions, and conducting post-marketing studies to evaluate the long-term safety and efficacy of the drug.
Role of Machine Learning & AI in the Pharma Drug Lifecycle:
ML algorithms can analyze large and complex datasets, identify patterns and trends, and make predictions or decisions based on this analysis. ML is a rapidly evolving field, with new techniques and algorithms being developed all the time and has the potential to transform the way we live and work.
How does ML solve the basic drug discovery problems?
The role of machine learning (ML) in drug discovery has become increasingly important in recent years. ML can be applied to various stages of the drug discovery process, from target identification to clinical trials, to improve the efficiency and success rate of drug development.
Stages of drug discovery process:
Phase | Goal |
Target Identification | Find all targets and eliminate wrong targets |
Lead discovery and optimization | Identify compounds and promising molecules |
Preclinical Development Stage | Eliminate molecules and analyze the safety of potential drug |
In the target identification stage, ML algorithms can analyze large-scale genomics and proteomics data to identify potential drug targets. This can help researchers identify novel targets that are associated with specific diseases and develop drugs that target these specific pathways.
In the lead discovery stage, ML can be used to screen large chemical libraries to identify compounds with potential therapeutic properties. ML algorithms can analyze the chemical structures and properties of known drugs and identify similar compounds that may have therapeutic potential. This can help accelerate the discovery of new drug candidates and reduce the time and cost of drug development.
In the lead optimization stage, ML can be used to predict the properties of potential drug candidates, such as their pharmacokinetics and toxicity, based on their chemical structures. This can help researchers prioritize and optimize the most promising compounds for further development, leading to more efficient drug development.
In the preclinical development stage, ML can be used to analyze the results of animal studies and predict the safety and efficacy of potential drug candidates in humans. This can help identify potential safety issues early in the development process and reduce the risk of adverse effects in human trials.
Advancements in Clinical Trials and Drug Safety with Machine Learning (ML)
Applications of ML in Clinical Trials:
ML algorithms can be used to optimize the design and execution of clinical trials. They can analyze patient data and identify suitable participants based on specific criteria, leading to more efficient and targeted recruitment. ML can also assist in patient stratification, helping researchers identify subpopulations that may respond better to the drug being tested. Furthermore, ML algorithms can analyze clinical trial data to predict patient outcomes, assess treatment response, and detect potential adverse effects.
ML in Drug Safety Assessment:
ML techniques can aid in the analysis of large datasets to identify patterns and detect safety signals associated with drug usage. By analyzing real-world data, including electronic health records and post-marketing surveillance data, ML algorithms can help identify potential adverse reactions and drug-drug interactions. This information can contribute to improving drug safety monitoring and post-market surveillance efforts.
Connections with Computer-Aided Drug Design (CADD) and Structure-Based Drug Design:
ML is closely related to CADD and structure-based drug design methodologies. CADD utilizes ML algorithms to analyze chemical structures, predict compound properties, and assess their potential as drug candidates. ML can also assist in virtual screening, where large chemical libraries are screened computationally to identify molecules with desired properties. Furthermore, ML can be employed to model protein structures and predict protein-ligand interactions, aiding in the design of new drug candidates.
How is AI/ML currently being applied in the pharmaceutical industry?
Drug Discovery:
AI/ML algorithms can identify potential drug targets, predict drug efficacy, toxicity, and side effects, which can reduce the time and cost of drug discovery. ML algorithms can analyze vast amounts of data, including gene expression, molecular structure, and biological pathway information, to generate new hypotheses about drug targets and drug interactions. Furthermore, AI/ML can predict which drug candidates have the best chances of success, increasing the likelihood of approval by regulatory agencies.
Clinical Trial Optimization:
AI/ML can help optimize clinical trials by identifying suitable patient populations, predicting treatment response, and identifying potential adverse events. By analyzing patient data, including clinical data, genomic data, and real-world data, AI/ML can identify subpopulations that are more likely to benefit from the drug and optimize the dosing and administration of the drug. Moreover, AI/ML can identify potential adverse events that may have been overlooked in traditional clinical trial designs.
Precision Medicine:
AI/ML can be used to analyze patient data, such as genomic, proteomic, and clinical data, to identify personalized treatment options based on individual patient characteristics. AI/ML can help identify genetic variations that may affect the efficacy or toxicity of a drug, leading to more targeted and personalized treatments. For instance, ML algorithms can analyze patient data and predict which patients are more likely to benefit from immunotherapy treatment for cancer.
Real-world Data Analysis:
AI/ML can be used to analyze large amounts of real-world data, such as electronic health records and claims data, to identify patterns and insights that can inform drug development and patient care. For example, AI/ML can help identify the causes of adverse events, such as drug-drug interactions, leading to better post-market surveillance and drug safety.
Drug Repurposing:
AI/ML can be used to identify existing drugs that can be repurposed for new indications, which can help reduce the time and cost of drug development. ML algorithms can analyze large amounts of data, including molecular structure, clinical trial data, and real-world data, to identify drugs that have the potential to treat a specific disease.
Imaging and Diagnosis:
AI/ML can be used to analyze medical images, such as CT scans and MRI scans, to improve diagnosis accuracy and speed. AI/ML algorithms can analyze large amounts of medical images and detect subtle changes that may be missed by human radiologists. For instance, AI/ML can analyze medical images and identify early signs of Alzheimer's disease or heart disease.
Predictive Maintenance:
AI/ML can be used to monitor equipment and predict when maintenance is needed, which can help reduce downtime and improve efficiency. ML algorithms can analyze data from sensors and predict when equipment is likely to fail, leading to more efficient maintenance and reduced downtime.
Some examples of the used AI and ML technology in the pharmaceutical industry
Tools | Details | Website URL |
---|---|---|
DeepChem | MLP model that uses a python-based AI system to find a suitable candidate in drug discovery | https://github.com/deepchem/deepchem |
DeepTox | Software that predicts the toxicity of total of 12,000 drugs | www.bioinf.jku.at/research/DeepTox |
DeepNeuralNetQSAR | Python-based system driven by computational tools that aid detection of the molecular activity of compounds | |
Organic | A molecular generation tool that helps to create molecules with desired properties | https://github.com/aspuru-guzik-group/ORGANI |
PotentialNet | Uses NNs to predict binding affinity of ligands | https://pubs.acs.org/doi/full/10.1021/acscentsci.8b00507 |
Hit Dexter | ML technique to predict molecules that might respond to biochemical assays | http://hitdexter2.zbh.uni-hamburg.de |
DeltaVina | A scoring function for rescoring drug–ligand binding affinity | https://github.com/chengwang88/deltavina |
Neural graph fingerprint | Helps to predict properties of novel molecules | https://github.com/HIPS/neural-fingerprint |
AlphaFold | Predicts 3D structures of proteins | https://deepmind.com/blog/alphafold |
Chemputer | Helps to report procedure for chemical synthesis in standardized format | https://zenodo.org/record/1481731 |
These examples demonstrate the application of AI and ML in different stages of the pharmaceutical drug lifecycle, from drug discovery to safety assessment and protein structure prediction.
Use cases of AI/ML Technology in Pharmaceutical Industry
- Protein Folding: AI and ML algorithms can predict the way proteins will fold, which is critical in drug discovery. For example, Google's DeepMind used AI to solve a 50-year-old problem in protein folding, which could lead to the development of new drugs.
- Adverse Event Detection: AI and ML algorithms can analyze patient data and detect adverse events associated with medications. For example, IBM Watson Health uses AI to detect adverse events in real-time, which can improve patient safety and help pharmaceutical companies respond quickly to potential issues.
- Supply Chain Optimization: AI and ML algorithms can optimize supply chain processes, such as demand forecasting and inventory management. For example, Merck uses AI to predict demand for their products and optimize their supply chain operations.
- Quality Control: AI and ML algorithms can be used to monitor manufacturing processes and detect defects or quality issues in real-time. For example, Novartis uses AI to monitor their manufacturing processes and detect potential quality issues before they become major problems.
- Drug Pricing: AI and ML algorithms can analyze market data and predict the optimal price for drugs. For example, Sanofi uses AI to optimize drug pricing based on factors such as market demand, patient access, and the cost of production.
AI/ML has become an essential tool in the pharmaceutical industry and R&D. The use of AI/ML can accelerate drug discovery, optimize clinical trials, personalize treatments, and improve patient outcomes. Moreover, AI/ML can analyze large amounts of data and identify patterns and insights that may have been missed by traditional methods, leading to better drug development and patient care. The future of AI/ML in pharma and R&D is promising, and it is expected to revolutionize the industry and improve patient outcomes.