
The problem with Data.. more we solve more it goes back to the same place
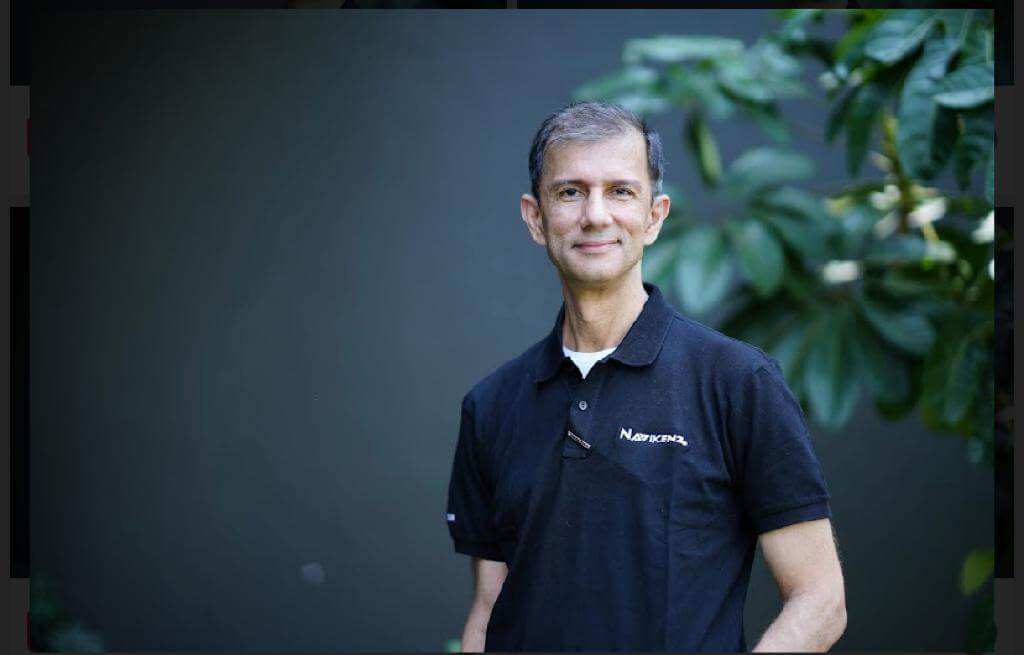
Anjan Lahiri
CEO
150 years ago Jean-Baptiste Alhonse Karr wrote “plus ca change, plus c’est la même chose – the more things change, the more they stay the same…
And so it is with our attempt to put our arms around Enterprise Data in an organization. We go from Data Balkanization to Data Centralization back to Data Balkanization with each iteration promising nirvana but finally realizing that things are back to where we started but faster and with greater ability to know for sure that we are not where we exactly wanted to be.
Data in the 1970/80’s was in ‘flat files’ written by COBOL programs. These were good for running operational applications but completely inaccessible when it came to ‘analysis’. Also each application had its own ‘Data’ in its own ‘files’. So in the 1990’s we moved all these disparate sources of inaccessible data files into huge monolithic ‘Data Warehouses’.
All data from the flat files was poured into structured formats into these Data Warehouses. All the enterprise data was now available ‘in one place’. Except that it was managed by the IT team, with very rigid rules and the sheer size and complexity meant that each time the business wanted any query, they had to wait in queue. Unfortunately, businesses do not run at the speed at which IT (in those days) could react and business domains started creating their own individual small Data Warehouses which were ‘Domain Specific’ called ‘Data Marts’.
These Data Marts were very efficient in serving the needs of the business domain like ‘Sales’, ‘Production’ or ‘Marketing’, but two big problems arose. One is that Enterprise Data started going out of sync. So a Customer name in the Sales Data Mart was now different from the Customer Name in Production and hence there was no way to get a unified view of the Customer, the Product, Sales or anything. So then people started putting complex rules of governance to define ‘Who is a Customer’ and ‘What is a Product’ to be followed by all individual ‘Domains’. But once again business will move at a higher speed than what rules can do to tie it down. The other problem was that while all the above was to do with ‘Structured Data’, it left out the fastest growing volume of new Data which was ‘Unstructured Data’. A Customer ID number with a Customer Name is structured Data. A tweet from a customer complaining about a product is unstructured data but one that just as critically important. So organizations needed to solve for both of the above problems.
Came the Data Lake. Into Data Lakes, just as in the Data Warehouses of old, all the Enterprise Data was poured in, both Structured and Unstructured giving one monolithic place where all the organizational data could be accessed.
We were back to where we started – a monolithic data repository managed by IT which could not be accessed by the business with the ease and speed required.
And by this time Cloud architecture was already there, with scalability and access to storage becoming a non issue. And that has brought us to the latest in this iteration with the Data Mesh architecture, where once again, just like the Data Marts of old, individual business domains are creating their own view of the Data. And thanks to the opportunities afforded by Cloud architecture, unlimited Storage and superfast Computing Speeds, a centralized governance infrastructure of rules surrounding who owns which Data and how to access Data across lines of ownership without having to go back to IT to make massive changes.
That is now a game changer bringing us back to the Balkanized view of data but with a common governance.
So coming up with the Data Architecture is the critical step for an organization that wants to be Digital Leader. We will not solve all the problems, but we will keep going back to the same place but with greater awareness.
What is the right Data Architecture for my organization that will enable me to harness the power of data completely to improve my Digital Maturity. That is the key question for everybody.